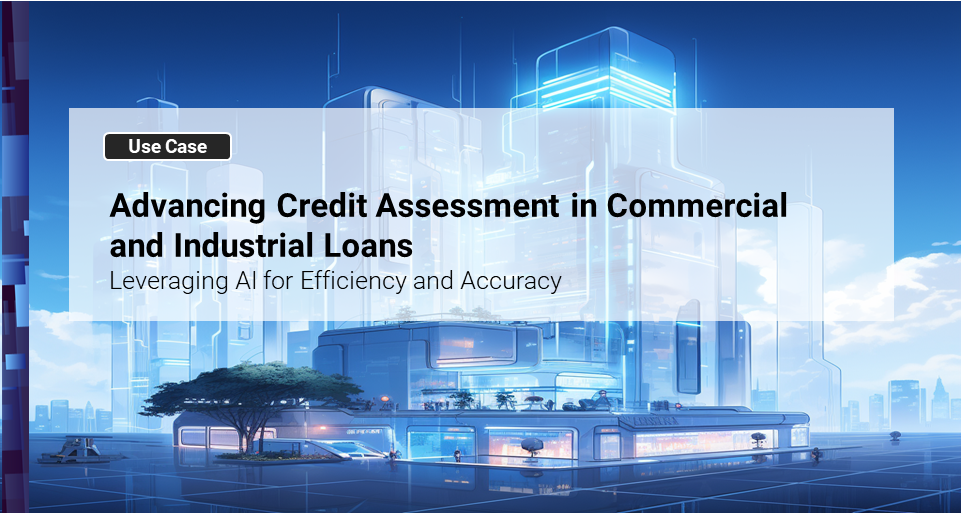
Background
In the fast-paced world of commercial and industrial lending, banks face the ongoing challenge of efficiently and accurately assessing the creditworthiness of companies. The traditional credit assessment process involves meticulous manual work, requiring extensive analysis of financial statements, business models, industry dynamics, credit histories, collateral, and compliance postures.
Credit and lending products, on average, take up more than 70% of commercial banks’ revenues. Banks analyze various financial and non-financial data before approving corporate loans. These credit-related data may be sourced from traditional and alternative sources. They may vary in type depending on the sector classification of the borrower, leading to the majority of data being reported in multiple formats. Credit analysts and relationship managers then use the credit-related data to generate credit reports for subsequent credit review and documentation. The unstructured nature of credit-related data hinders a bank’s ability to efficiently evaluate a company’s creditworthiness or benchmark against previous credit records – leading to lengthy credit processing lead time and increased operational risks.
As a result, many banks have begun exploring transformative technologies to address the following pain points throughout the credit assessment process:
- Speed – Long lead times in the credit approval process can hinder customer experience and limit the amount of credit a bank can distribute. Increasingly, qualified and high-quality borrowers seek reliable and trustworthy banking partners to channel their funds more efficiently and rapidly, making speed-to-approval a top priority for financial institutions.
- Accessibility – Historical data may have substantial analytical value but is not easily accessible as they are stored in unstructured formats. Without AI, it is challenging for a bank to easily search and categorize data based on various criteria. The lack of digitized and structured precedents hinders the bank’s ability to reference previous credit decisions and benchmark companies.
- Data Risk – Data collected during the credit assessment may vary across sectors and can be analyzed in siloes by various teams, leading to potential blindspots throughout the lending cycle. As data is manually gathered, segmented, and stored, human errors can potentially occur.
In most banks, credit documents can have up to several hundred pages, are unstructured, may contain multiple languages, and are stored in various formats, including PDF, Word, RTF, Excel, and more. For every review, the credit team must manually extract relevant information, including key parties, locations, segments, financials, and more, to evaluate the borrower’s creditworthiness.
Applying new data sources, advanced analytics, and machine learning technologies can streamline processes, increase the accuracy of risk models, and enable banks to make holistic and data-driven decisions. However, most financial institutions lack the necessary capabilities, such as infrastructure and talent, to effectively implement artificial intelligence in existing processes. This creates an opportunity for technology providers to design no-code solutions to enable traditional banks to leverage the latest technologies.
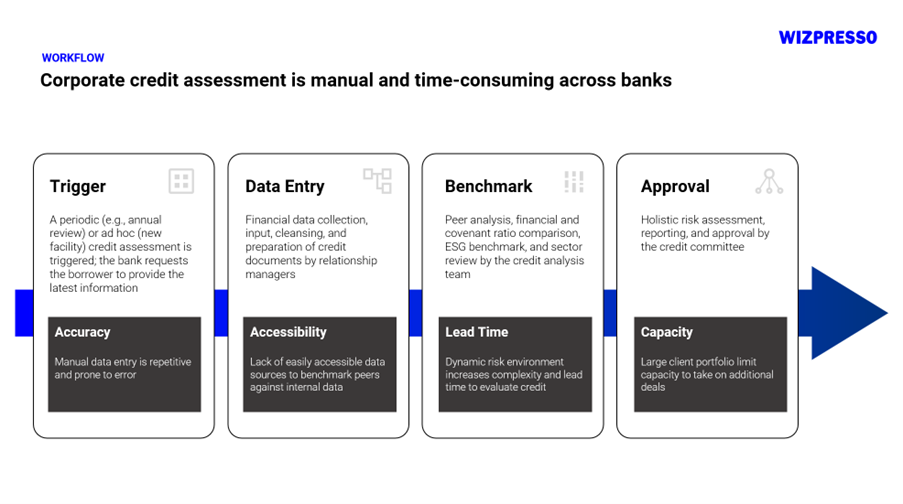
Wizpresso Credit Assessment Solution powered by Adnoto
Adnoto is a no-code knowledge management software with AI-powered document intelligence capabilities. The software leverages the following technologies and can be deployed on-premise or on the cloud based on the client’s cybersecurity requirements:
- Our prebuilt user interface enables relationship managers to seamlessly manage transactions, upload financial statements and supporting documents to prepare a credit report.
- Our pretrained AI models can efficiently extract data from financial statements, data tables, text, and forms and parse them into a structured format.
- Users can create customized models using no-code to perform automated analysis, such as pre-screening, sentiment analysis, and ratio comparison.
- Financial and ESG performance indicators can be benchmarked with data from our database of 25,000+ publicly listed companies across 12 capital markets.
- Structured data can be exported using an API and integrated with other systems
- Relationship managers can utilize our document management system to organize and curate client-submitted documents.
- Our software undergoes robust code scans and penetration tests. All data is encrypted at rest and in transit using industry best practices.
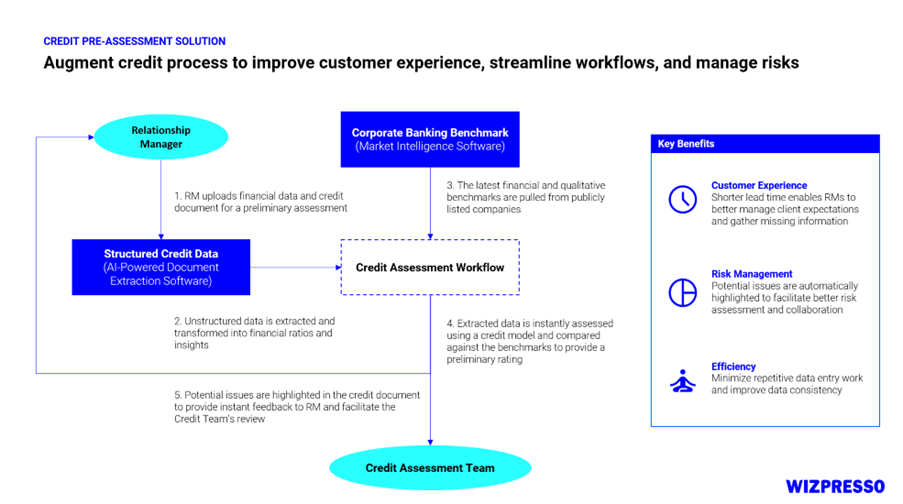
Conclusion
Credit assessment is a complex process that involves thoroughly reviewing forms, KYC documents, financials, and supporting information. Traditionally, this process has relied heavily on manual work, which limits the banks’ ability to leverage their existing knowledge base and accelerate dealmaking. With the advancement of AI technology, banks can enhance their credit assessment processes, improve efficiency, and make informed transaction decisions.
Learn more about Adnoto
Product Page: https://wizpresso.com/KnowledgeManagement/Adnoto